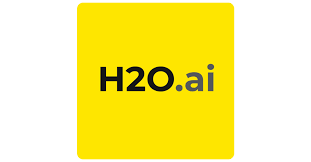
H2O Danube 3 is the latest iteration in the H2O.ai's series of small language models (SLMs), designed to provide powerful natural language processing capabilities in a compact and efficient package. This article delves into the technical details, benchmarks, and optimal use cases for H2O Danube 3, showcasing its potential as a versatile tool for various AI applications.
Model Architecture and Specifications
H2O Danube 3 is a family of decoder-only language models that come in different sizes to cater to various computational requirements:
- H2O-Danube3-4B: The flagship model with 4 billion parameters
- H2O-Danube3-500M: A smaller variant with 500 million parameters
Both models utilize a state-of-the-art architecture that builds upon the success of their predecessors, incorporating several key improvements:
- Attention Mechanism: The sliding window approach for attention has been removed, enhancing long-context behavior and improving retrieval capabilities.
- Training Data: The models have been trained on a vast corpus of text, with the 4B version likely trained on even more data than its smaller counterpart.
- Context Window: H2O Danube 3 models support a context window of 8,192 tokens, allowing for processing of longer text sequences.
Benchmarks and Performance
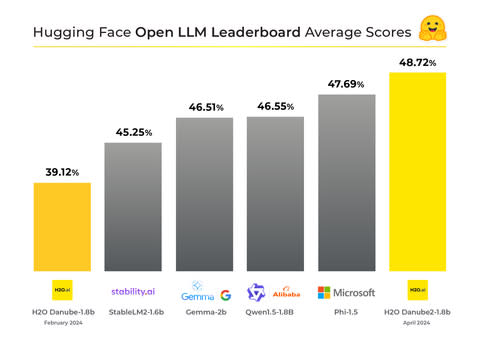
H2O Danube 3 has demonstrated impressive performance across a wide range of benchmarks, particularly for its parameter count. Let's examine the results for both the 4B and 500M variants:
H2O-Danube3-4B Benchmarks
The 4B model shows competitive results across various academic benchmarks, often ranking among the top performers in its class. Here's a comparison with other similar-sized models:
Benchmark | Metric | H2O-Danube3-4B | Qwen1.5-4B | StableLM-3B | Phi-3-mini-4B |
---|---|---|---|---|---|
ARC-c | 25-shot | 58.96 | 42.15 | 47.70 | 63.91 |
Hellaswag | 10-shot | 80.36 | 69.46 | 73.71 | 80.62 |
MMLU | 5-shot | 54.74 | 54.03 | 44.98 | 69.43 |
TruthfulQA | 0-shot mc2 | 47.79 | 44.88 | 46.40 | 57.72 |
Winogrande | 5-shot | 76.48 | 66.22 | 65.59 | 70.80 |
GSM8K | 5-shot | 50.18 | 3.63 | 52.46 | 77.48 |
CommonsenseQA | 3-shot | 79.52 | 76.09 | 75.76 | 77.81 |
Average | - | 68.98 | 57.07 | 63.21 | 76.01 |
Key observations:
- H2O-Danube3-4B achieves the highest score in the CommonsenseQA benchmark, showcasing its strong common-sense reasoning abilities.
- It performs exceptionally well on the Hellaswag benchmark, with a score of 80.36%, approaching the performance of much larger models.
- The model demonstrates balanced performance across various tasks, including reasoning, knowledge-based questions, and language understanding.
H2O-Danube3-500M Benchmarks
The 500M variant also shows impressive results for its size, often outperforming similar-sized models:
Benchmark | Metric | H2O-Danube3-500M | Qwen2-0.5B |
---|---|---|---|
ARC-c | 25-shot | 39.25 | 32.00 |
Hellaswag | 10-shot | 67.53 | 61.37 |
MMLU | 5-shot | 36.57 | 34.97 |
TruthfulQA | 0-shot mc2 | 41.81 | 39.82 |
Winogrande | 5-shot | 63.13 | 60.85 |
The H2O-Danube3-500M model outperforms the Qwen2-0.5B model across all listed benchmarks, making it a strong contender in the sub-1B parameter category.
Optimal Use Cases for H2O Danube 3
H2O Danube 3 models are versatile and can be applied to a wide range of natural language processing tasks. Here are some scenarios where these models excel:
Retrieval-Augmented Generation (RAG): The improved long-context behavior makes H2O Danube 3 particularly suitable for RAG applications, where it can effectively process and generate responses based on large amounts of retrieved information.
Open-ended Text Generation: The models can generate coherent and contextually relevant text for various applications, such as content creation or creative writing assistance.
Summarization: With their strong language understanding capabilities, H2O Danube 3 models can effectively condense long documents into concise summaries.
Question Answering: The models perform well on knowledge-based benchmarks, making them suitable for building Q&A systems across various domains.
Chatbots and Conversational AI: The chat-tuned versions of H2O Danube 3 are optimized for dialogue-based interactions, making them ideal for building chatbots and conversational interfaces.
Data Formatting and Table Creation: The models can understand and generate structured data, making them useful for tasks involving data manipulation and presentation.
Paraphrasing and Rewriting: H2O Danube 3 can effectively rephrase and rewrite text while maintaining the original meaning, useful for content optimization and language learning applications.
Chain-of-Thought Reasoning: The models' strong performance on reasoning tasks makes them suitable for applications requiring step-by-step problem-solving or logical deduction.
Information Extraction: H2O Danube 3 can be used to extract relevant information from unstructured text, aiding in data mining and analysis tasks.
Fine-tuning for Specific Domains: The base models can be further fine-tuned on domain-specific data to create specialized models for industries such as healthcare, finance, or legal.
For more details, you can read the H2O Danbu 3 Technical Report:

Here's our summary of the report:
Advantages of H2O Danube 3
Efficiency: As small language models, H2O Danube 3 variants require fewer computational resources than larger models, making them more cost-effective and easier to deploy.
Versatility: The models perform well across a wide range of tasks, making them suitable for diverse applications without the need for multiple specialized models.
Open-source: H2O Danube 3 models are released under the Apache 2.0 license, allowing for free use, modification, and distribution.
Privacy-friendly: The smaller size of these models enables on-device or on-premises deployment, reducing the need to send sensitive data to external servers.
Customizability: The base models can be easily fine-tuned for specific tasks or domains, allowing for tailored solutions.
Limitations and Considerations
While H2O Danube 3 models offer impressive capabilities, it's important to consider their limitations:
Parameter Count: Although highly efficient, these models may not match the performance of much larger models (100B+ parameters) on certain complex tasks.
Specialized Knowledge: For highly specialized domains, additional fine-tuning or domain-specific training may be necessary to achieve optimal performance.
Ethical Considerations: As with all AI models, users should be aware of potential biases and implement appropriate safeguards when deploying H2O Danube 3 in real-world applications.
Conclusion
H2O Danube 3 represents a significant advancement in the field of small language models, offering a compelling balance between performance and efficiency. With its strong benchmark results, versatility across various NLP tasks, and the ability to be fine-tuned for specific applications, H2O Danube 3 is poised to become a valuable tool for developers, researchers, and businesses looking to leverage the power of language AI without the overhead of massive models.
As the AI landscape continues to evolve, models like H2O Danube 3 play a crucial role in democratizing access to advanced language processing capabilities. By providing powerful, open-source solutions that can run on more modest hardware, H2O.ai is enabling a wider range of organizations and individuals to benefit from the latest advancements in natural language processing technology.
Whether you're building a chatbot, developing a content generation tool, or creating a domain-specific question-answering system, H2O Danube 3 offers a robust foundation that can be tailored to meet your specific needs. As the community continues to explore and expand upon these models, we can expect to see even more innovative applications and improvements in the future of small language models.
from Anakin Blog http://anakin.ai/blog/h2o-danube-3/
via IFTTT
No comments:
Post a Comment