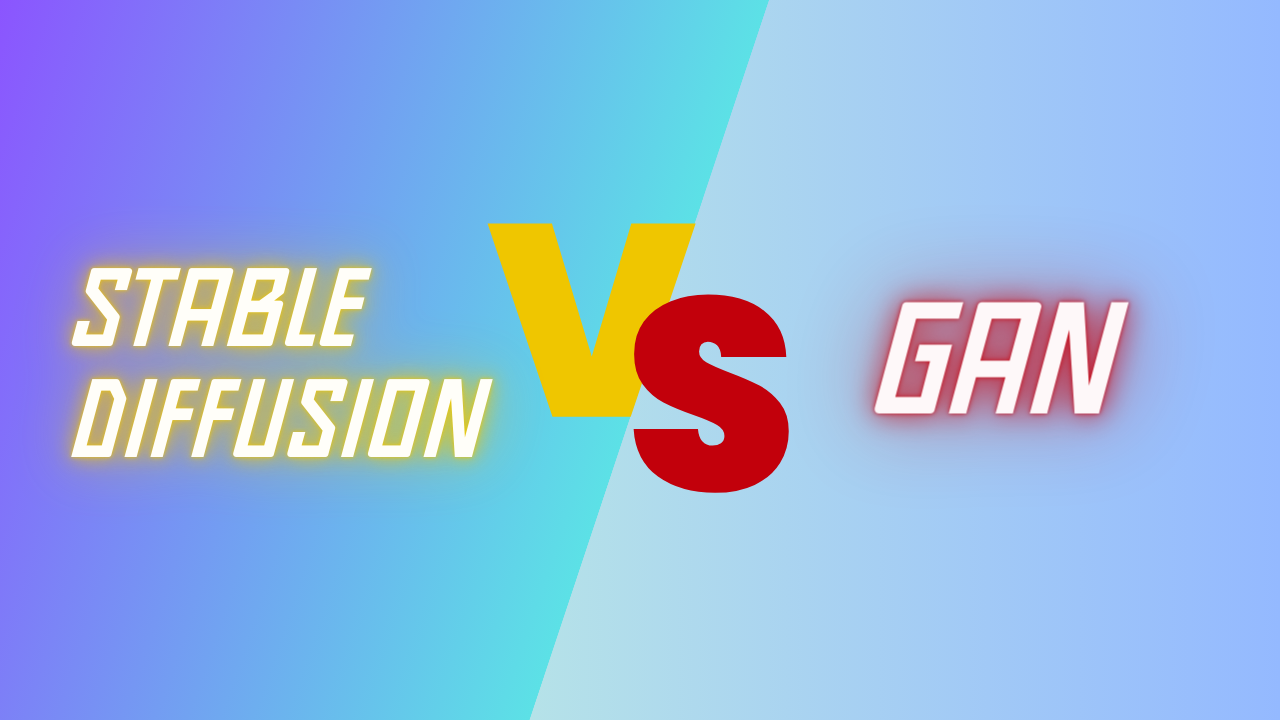
Generative AI has revolutionized the field of computer vision by enabling machines to create realistic images, videos, and even text. Among the various techniques used in generative AI, Generative Adversarial Networks (GANs) have been widely popular and successful. However, a new contender has emerged: Stable Diffusion. In this blog post, we will explore the battle between GANs and Stable Diffusion, comparing their strengths and weaknesses, and showcasing the power of Stable Diffusion using Anakin AI's Stable Diffusion Image Generator.
Article Summary
Comparing the available Generative Models such as: Autoencoders, GANs (Generative Adversarial Networks), and Diffusion Models.
Stable Diffusion Models are more popular among general usage, especially for creative art.
GANs are more useful in the case of Realistic Time Lapse Imagery.
What are Generative Models?
Generative models are deep learning models that have the ability to generate new samples that resemble the training data. These models learn the underlying distribution of the training data and can generate new samples that are similar to the observed data. They have been used in a wide range of applications, from creating realistic images to generating new music compositions.
Interested in Generative Models?
Don't forget that you can generate awesome images with Stable Diffusion online generator now!
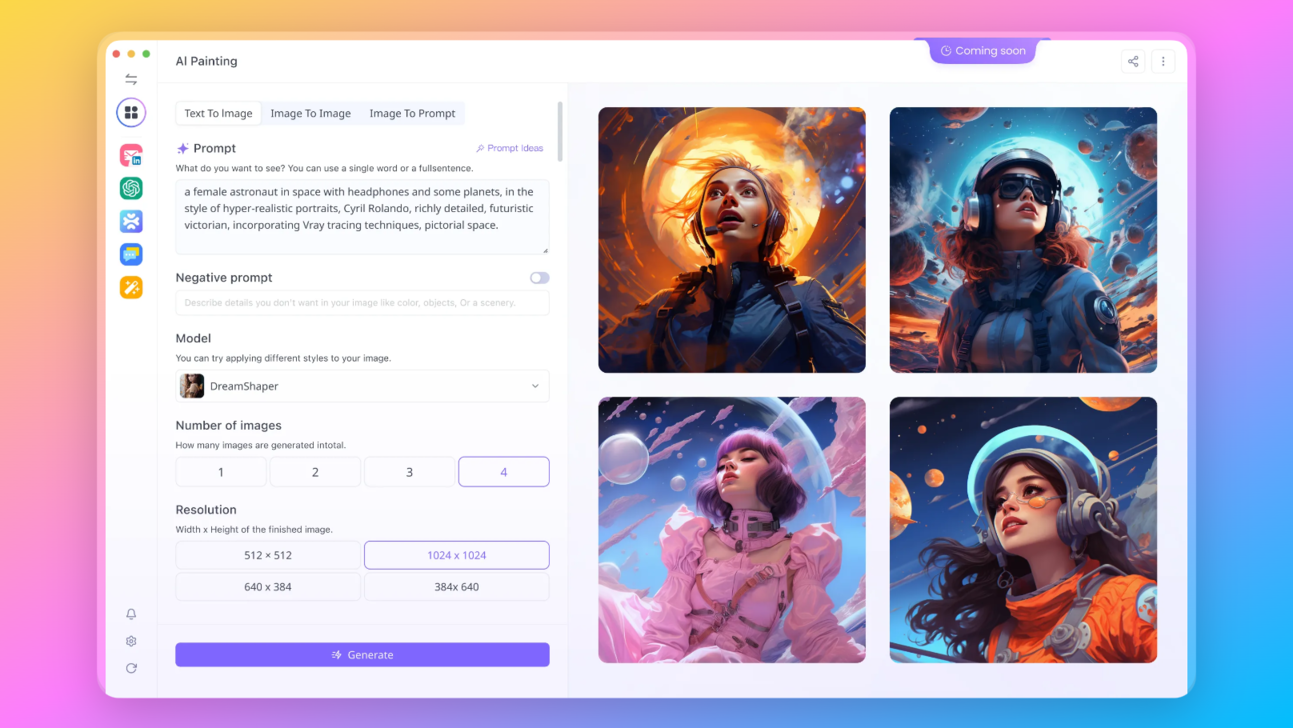
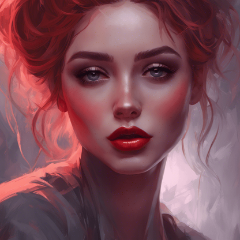
The History of Generative AI
Generative AI has come a long way since its inception. Early developments in generative models were limited in their ability to generate high-quality images. However, advancements in deep learning and computational power have led to the emergence of more sophisticated models that can generate incredibly realistic images, videos, and text.
Explosion of Generative AI Text-to-Image Models
One of the significant breakthroughs in generative AI was the development of text-to-image models. These models can generate images based on textual descriptions, allowing for a direct translation of text into visuals. This innovation has had a profound impact on creative industries such as advertising, gaming, and digital art.
Image Generation Models: A Comparison
Early image generation models faced several technical challenges such as mode collapse, lack of diversity, and difficulty in training. Researchers and developers have worked tirelessly to overcome these limitations, leading to improved models and techniques that can generate high-quality and diverse images.
Autoencoders
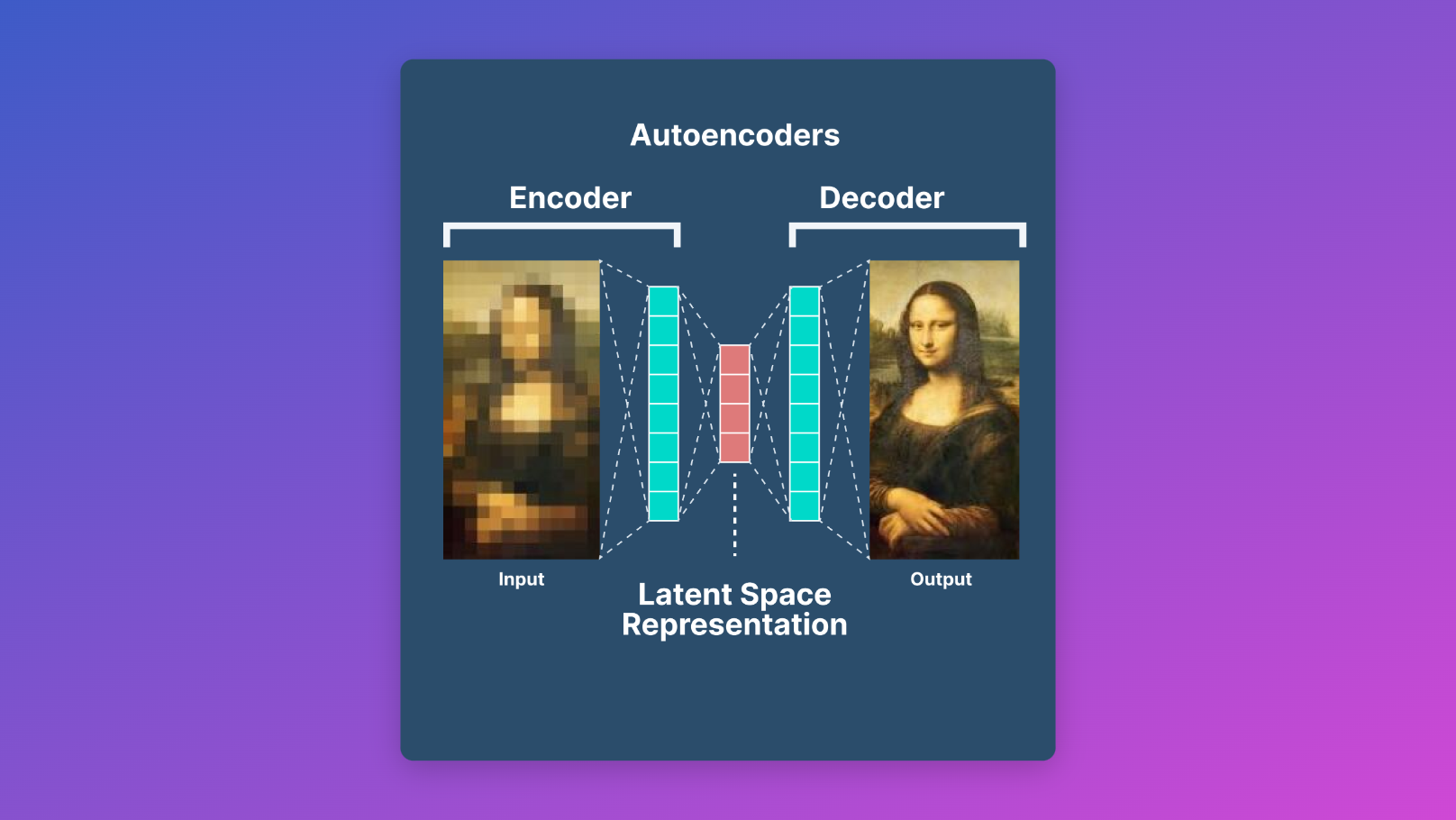
Autoencoders are a class of generative models that learn to encode and decode data. They are widely used in image generation tasks and have been instrumental in advancing the field of generative AI. Autoencoders have their strengths but also some limitations compared to other generative models.
GANs (Generative Adversarial Networks)
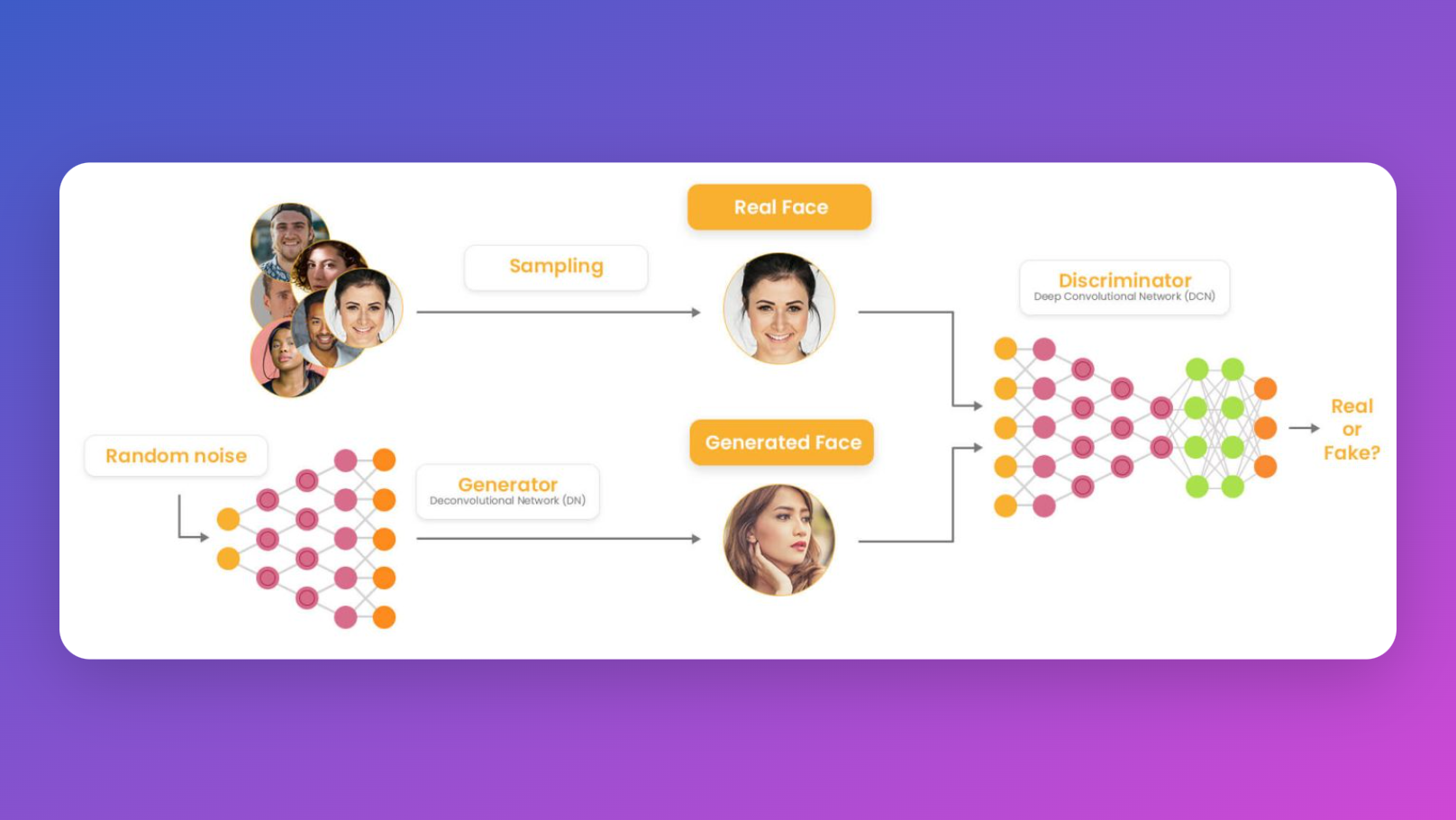
GANs are arguably the most well-known and widely used generative models in the field of computer vision. They consist of two main components: a generator and a discriminator. The generator tries to generate realistic images, while the discriminator tries to distinguish between real and generated images. GANs have been highly successful in generating high-quality images but come with their own set of limitations.
Diffusion Models
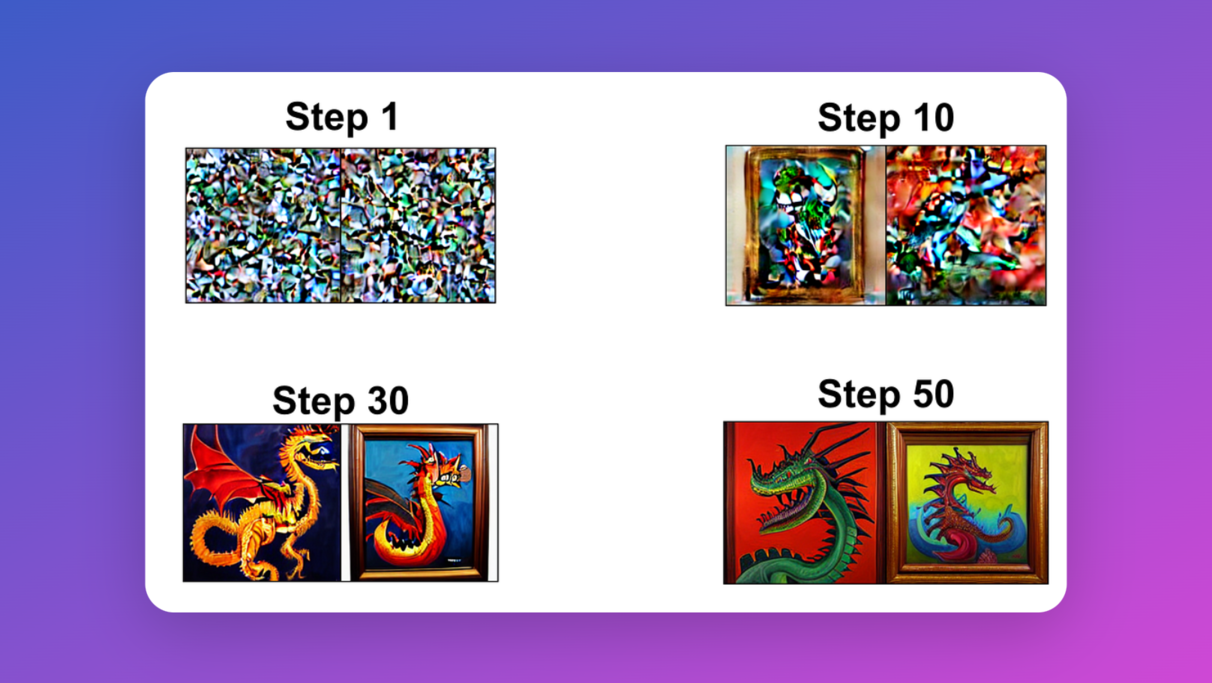
Diffusion models are a relatively new entrant in the field of generative AI but have gained attention for their unique approach. Unlike GANs, diffusion models generate images by iteratively refining a random noise input. This iterative process allows for the generation of high-quality images with impressive details and realism.
Stable Diffusion vs GAN: A Detailed Comparison
Now let's dive into a detailed comparison between Stable Diffusion and GANs, showcasing the strengths and weaknesses of each model. This analysis will help you understand which model is better suited for your specific image generation needs.
Training and Computational Requirements
- GANs: Historically, GANs were preferred for image generation, but they are challenging to train. Achieving a stable equilibrium between the generator and discriminator is complex, and they are prone to mode collapse, where the generator fails to produce diverse outputs.
- Diffusion Models: Compared to GANs, diffusion models like Stable Diffusion are simpler to train. The denoising process is reliable, allowing generation from noise through an iterative refinement process. However, they are computationally more demanding than GANs, especially in direct diffusion processes for high-resolution images. Latent diffusion models, which operate in the latent space of a Variational Autoencoder (VAE), address some of these computational challenges.
- Transformers: While often associated with text generation, transformers can also be applied to image models. They represent an architectural choice, different from the generative process choice in diffusion or GAN models. Autoregressive transformers for images and diffusion models for text have also shown promising results, indicating versatility.
Specific Use Cases
- Creative Variance: Diffusion models are ideal for generating creative images with significant variance, offering a rich palette for artistic and innovative outputs.
- Realistic Time Lapse Imagery: For generating time-lapse images, such as a plant growing, both diffusion models and GANs could be suitable. The diffusion process can be adapted to denoise one timestep into the next, effectively capturing the gradual growth. GANs could also be formulated to generate each timestep from the previous, although it might require more careful tuning to maintain consistency in the background.
Model Choice and Dataset Quality
- It's important to note that the choice of the model type (diffusion, GANs, autoregressive transformers) often depends more on the quality and size of the dataset than the inherent characteristics of the models themselves.
- Each model has its strengths, but the final output quality is greatly influenced by the input data. Therefore, selecting a model should also consider the availability and quality of the training data.
Bridging the Gap Between Models
- Recent research is exploring ways to bridge the gap between autoregression and diffusion models, viewing both as instances of time-dependent denoising processes. This research indicates potential future developments where the distinct advantages of each model type might be combined or leveraged in hybrid approaches.
Pros and Cons of Stable Diffusion
Strengths of Stable Diffusion:
Stable Diffusion offers several advantages over GANs, including:
- Stable training: Stable Diffusion models are easier to train compared to GANs, making them more accessible to a wider range of users.
- Consistent output quality: Stable Diffusion consistently generates high-quality images without the risk of mode collapse, a common issue in GANs.
- Better handling of complex image generation tasks: Diffusion models have shown better performance in generating images with complex textures, fine details, and sharp edges.
- Improved stability during training: Stable Diffusion models exhibit better training stability and convergence properties, reducing the chances of training failures.
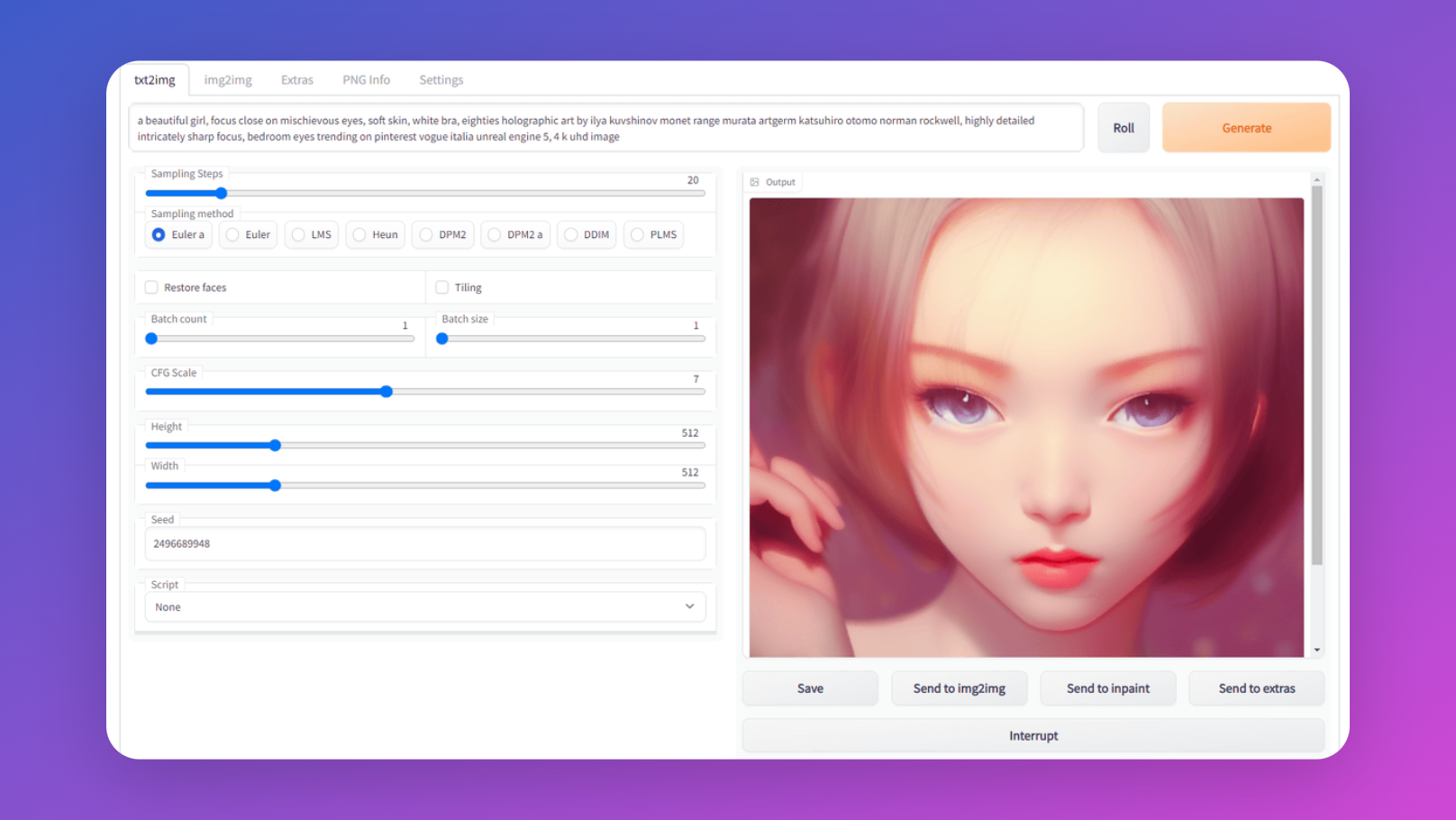
Cons of Stable Diffusion:
While Stable Diffusion has its strengths, it also has some limitations:
- Longer inference time: Generating images with Stable Diffusion takes more time compared to GANs, as it requires multiple iterations to refine the initial noise input.
- Higher computational requirements: Stable Diffusion models require more computational resources during training and inference compared to GANs.
- Limited applications: Stable Diffusion models are currently more suitable for image generation tasks and may not be as versatile as GANs for other applications such as image-to-image translation.
Use Cases and Performance Analysis
To understand the real-world performance of Stable Diffusion and GANs, it's essential to explore their use cases and evaluate their performance in various scenarios. This analysis will provide insights into which model is better suited for specific image generation tasks, such as generating landscapes, portraits, or abstract art.
The Future of Generative AI: Stable Diffusion or GAN?
Generative models, including both GANs and diffusion models, continue to evolve and improve. The future of generative AI holds exciting possibilities, such as more stable and efficient training algorithms, better handling of complex image generation tasks, and improved scalability. However, as generative AI becomes more powerful, it is crucial to address the ethical considerations and potential risks associated with its use.
Conclusion
In conclusion, the battle between GANs and Stable Diffusion continues to shape the field of generative AI. While GANs have dominated the landscape for a long time, Stable Diffusion shows great promise as a more stable and reliable alternative. With Anakin AI's Stable Diffusion Image Generator, you can unleash the power of Stable Diffusion and effortlessly create stunning images. Whether you choose GANs or Stable Diffusion, the future of generative AI holds tremendous potential for transforming creative industries and impacting various other domains. Start your journey into the world of generative AI today and unlock endless possibilities.
One more thign:
Try Anakin AI's Stable Diffusion Image Generator today and unleash the power of generative AI at your fingertips!
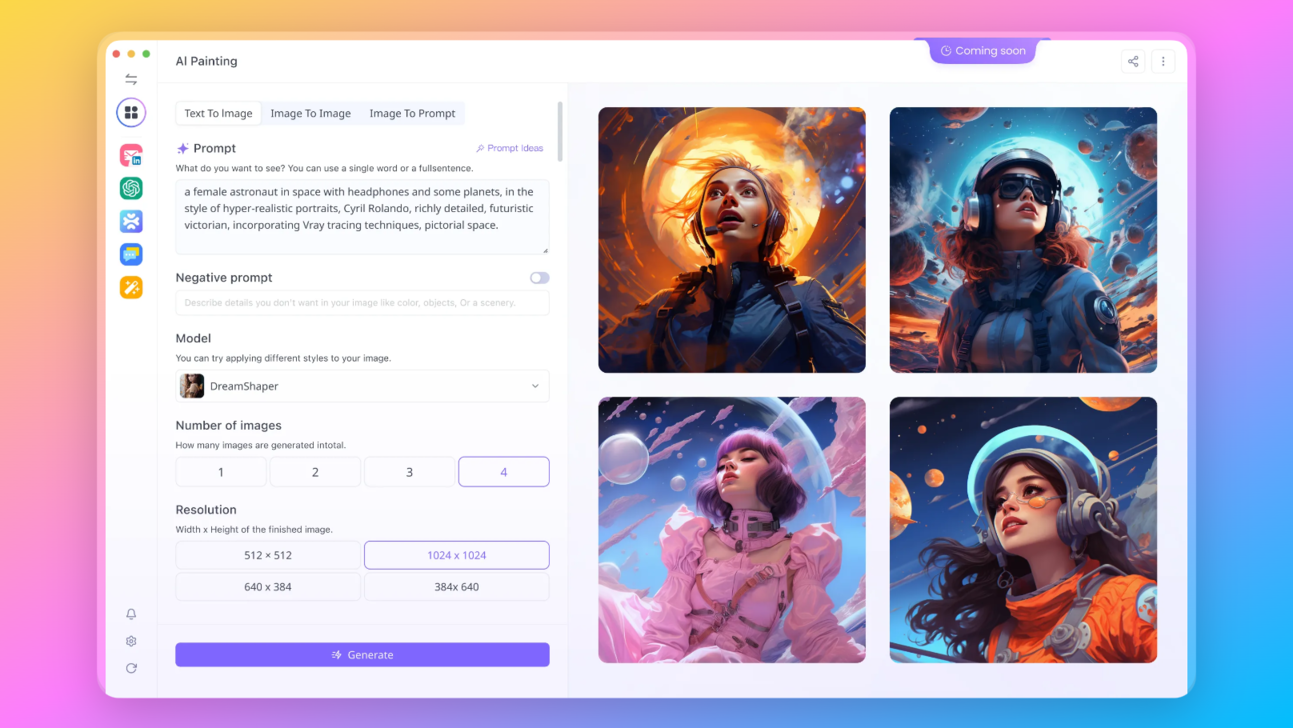
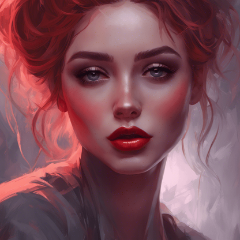
Create stunning images with ease using the most advanced diffusion model technology. Get started now at Anakin AI's Stable Diffusion Image Generator and transform your creative ideas into reality!
from Anakin Blog http://anakin.ai/blog/stable-diffusion-vs-gan/
via IFTTT
No comments:
Post a Comment