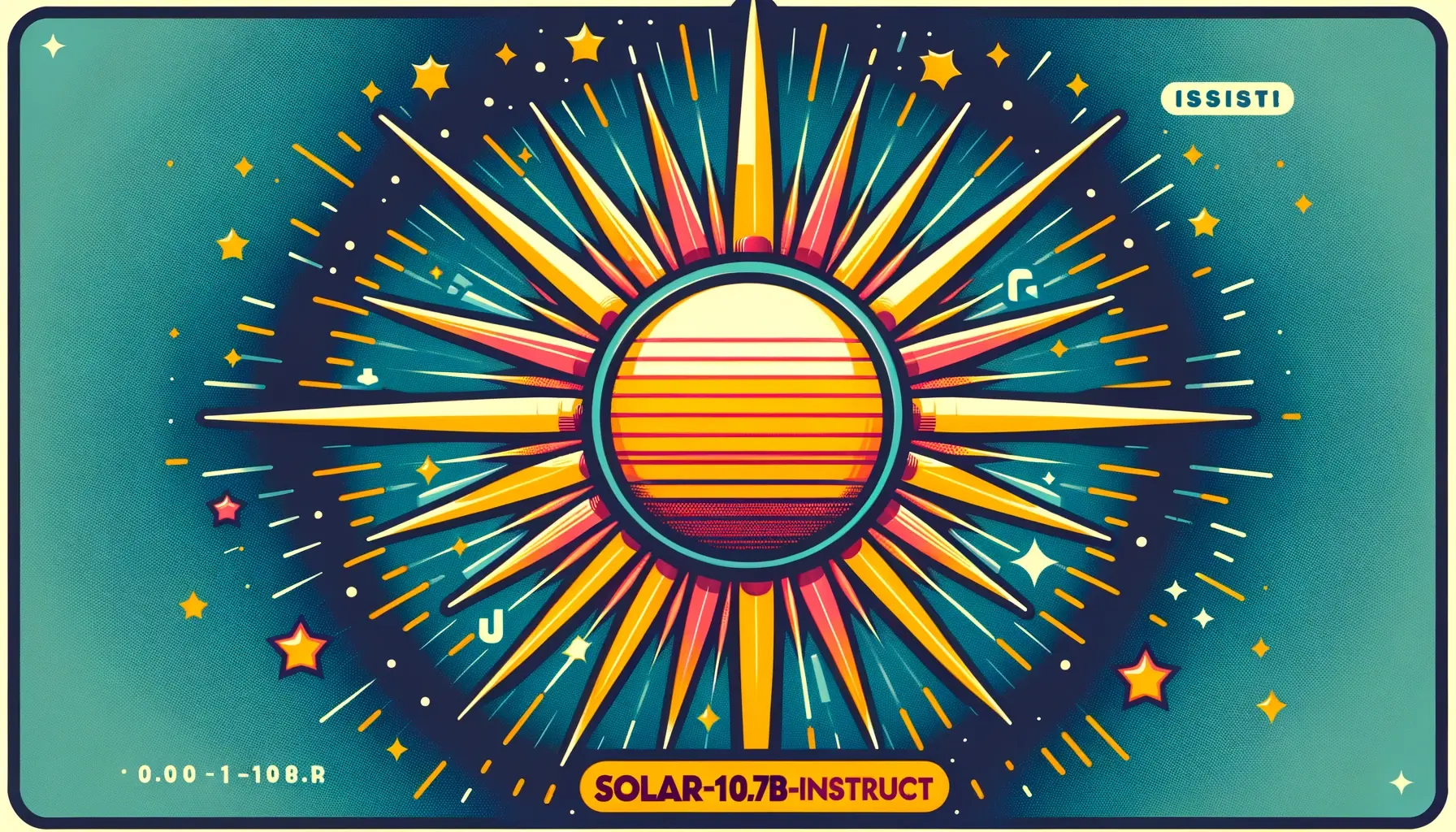
The landscape of artificial intelligence and natural language processing has witnessed a remarkable breakthrough with the introduction of SOLAR-10.7B-Instruct-v1.0 by Upstage. This cutting-edge model is not just a step forward in language modeling; it's a giant leap, showcasing unparalleled capabilities with its 10.7 billion parameters. We will discuss the technical details for the SOLAR-10.7B-Instruct-v1.0 model in the following passage.
Key Highlights
- Innovative Architecture: Leveraging the Depth Up-Scaling technique fused with the Llama2 architecture.
- Superior Performance: Outperforming models with up to 30 billion parameters, including the notable Mixtral 8X7B.
- Specialized Fine-Tuning: Excelling in single-turn conversations, SOLAR-10.7B-Instruct-v1.0 sets a new bar for precision and efficiency.
How Does SOLAR-10.7B-Instruct Work?
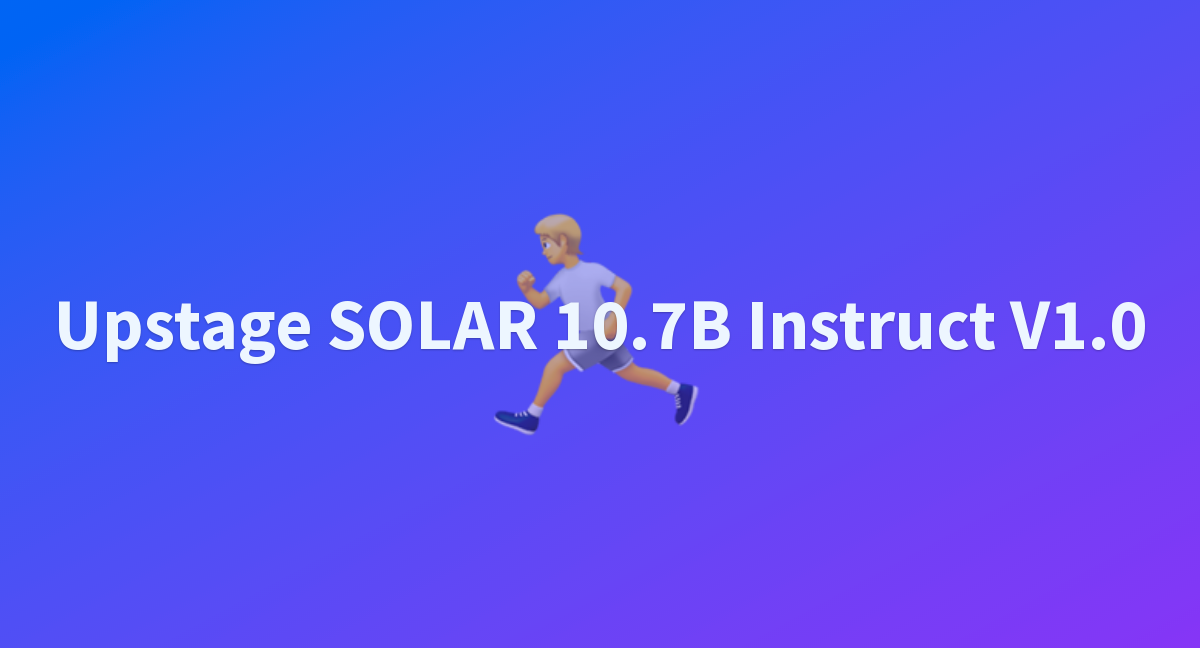
- SOLAR-10.7B-Instruct-v1.0 is highly proficient in following instructions, even to the point of incorporating excessive detail into its responses. This precision, while remarkable, requires careful prompting to manage the level of detail in responses.
- SOLAR-10.7B-Instruct-v1.0 writing style is very formal and complex.
- SOLAR-10.7B-Instruct-v1.0 is created by merging with Mistral 7B weights , which enhances its learning capacity, allowing it to assimilate and process information more effectively.
- The model’s foundation in the Llama2 architecture ensures a blend of speed and accuracy, making it a formidable tool in language processing.
Depth Up-Scaling: A Game-Changer?
SOLAR-10.7B introduces the revolutionary Depth Up-Scaling technique, setting it apart from conventional language models. This technique involves enhancing the depth of the model's neural network layers without a proportional increase in computational demands. This innovation not only improves the model's efficiency but also significantly boosts its performance.
Key Aspects of Depth Up-Scaling:
- Integration with Mistral 7B Weights:
- Built on Llama2 Architecture:
- Continued Pre-Training: Post-integration, the model undergoes extensive pre-training, further refining its abilities and adaptability.
The result of these combined efforts is a model that not only matches but often surpasses the performance of models with significantly larger parameter counts. SOLAR-10.7B is thus an ideal candidate for various fine-tuning applications, given its robustness and adaptability.
SOLAR-10.7B Benchmarks: Matches the Hype?
Data Contamination Test Results
In an era where data integrity is paramount, SOLAR-10.7B-Instruct-v1.0 stands out with its rigorous data contamination testing. Ensuring the model's training is free from benchmark-related datasets is crucial for its authenticity and reliability.
Model | ARC | MMLU | TruthfulQA | GSM8K |
---|---|---|---|---|
SOLAR-10.7B-Instruct-v1.0 | result < 0.1, %: 0.06 | result < 0.1, %: 0.15 | result < 0.1, %: 0.28 | result < 0.1, %: 0.70 |
These results indicate the model's high fidelity, with contamination rates well below concerning thresholds. This careful approach to training ensures that SOLAR-10.7B-Instruct-v1.0 delivers genuine and reliable outputs.
Evaluation Results
To further establish its efficacy, SOLAR-10.7B-Instruct-v1.0 underwent extensive evaluation against other leading models. The evaluation focused on several key areas, including the Model H6 score, which reflects the model's proficiency in understanding and responding to complex instructions.
Model | H6 | Model Size |
---|---|---|
SOLAR-10.7B-Instruct-v1.0 | 74.20 | ~ 11B |
mistralai/Mixtral-8x7B-Instruct-v0.1 | 72.62 | ~ 46.7B |
01-ai/Yi-34B-200K | 70.81 | ~ 34B |
01-ai/Yi-34B | 69.42 | ~ 34B |
mistralai/Mixtral-8x7B-v0.1 | 68.42 | ~ 46.7B |
meta-llama/Llama-2-70b-hf | 67.87 | ~ 70B |
tiiuae/falcon-180B | 67.85 | ~ 180B |
SOLAR-10.7B-v1.0 | 66.04 | ~ 11B |
mistralai/Mistral-7B-Instruct-v0.2 | 65.71 | ~ 7B |
Qwen/Qwen-14B | 65.86 | ~ 14B |
01-ai/Yi-34B-Chat | 65.32 | ~ 34B |
meta-llama/Llama-2-70b-chat-hf | 62.40 | ~ 70B |
mistralai/Mistral-7B-v0.1 | 60.97 | ~ 7B |
mistralai/Mistral-7B-Instruct-v0.1 | 54.96 | ~ 7B |
This evaluation underscores SOLAR-10.7B-Instruct-v1.0’s impressive capabilities in handling complex language tasks, especially in comparison to larger models. Its high H6 score is a testament to its advanced understanding and processing abilities, setting a new standard in the field of language modeling.
Advanced Training and Fine-Tuning Strategies
SOLAR-10.7B-Instruct-v1.0’s exceptional performance can be attributed to its advanced training and fine-tuning strategies. These strategies are designed to optimize the model’s ability to understand and respond to a wide range of instructions and queries with remarkable accuracy.
Key Strategies in Model Development:
- Supervised Fine-Tuning (SFT): This approach involves training the model on carefully curated datasets to enhance its response accuracy.
- Direct Preference Optimization (DPO): DPO focuses on aligning the model's outputs with user preferences, ensuring responses are not only accurate but also relevant to the user's needs.
- Use of Diverse Datasets: The model has been trained using a mixture of datasets, including in-house generated data and publicly available datasets, ensuring a comprehensive learning experience.
Ensuring Data Integrity:
- Data Contamination Avoidance: Great care was taken to prevent data contamination, especially from benchmark-related datasets, to maintain the model's integrity and reliability.
- Filtering Tasks: Specific tasks were filtered out during training to avoid overfitting and bias in the model's responses.
The combination of these sophisticated training methods and the rigorous avoidance of data contamination has culminated in a model that sets new benchmarks in the field of language processing.
Looking Forward: The Future of Language Modeling
The introduction of SOLAR-10.7B-Instruct-v1.0 marks a significant milestone in the journey of natural language processing and artificial intelligence. Its advanced architecture and training strategies have opened new avenues for research and application, from enhancing conversational AI to providing sophisticated language analysis tools.
Implications for Future Developments:
- Setting New Standards: SOLAR-10.7B-Instruct-v1.0 raises the bar for what is possible in language modeling, challenging other models and developers to push the boundaries further.
- Potential for Diverse Applications: Given its adaptability and robustness, this model can be fine-tuned for a wide range of applications, from customer service chatbots to advanced research in linguistics and AI.
Model Compatibility and Performance in Specific Tasks
- Discussions around the model's compatibility with existing tools and libraries, like Llama cpp and GGUF, were prominent, indicating a keen interest in practical applications and ease of use.
- Some users on Reddit conducted personal benchmarks, reporting that the model performs well in single-turn conversations but may struggle with multi-turn interactions.
Concerns About Data Contamination and Benchmark Gaming
- There were concerns about whether SOLAR-10.7B-Instruct-v1.0's training data included leaderboard test data, which could skew its performance metrics.
- Community members expressed wariness about models claiming high performance based on potentially biased benchmarks.
Want to test out all these awesome LLMs online? Try Anakin AI!
Anakin AI is one of the most convenient way that you can test out some of the most popular AI Models without Here are the other Open Source and Free Models that Anakin AI supports:
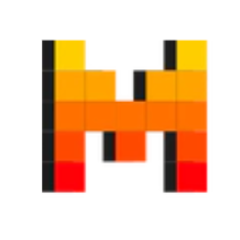
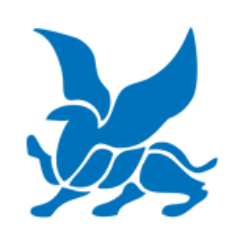
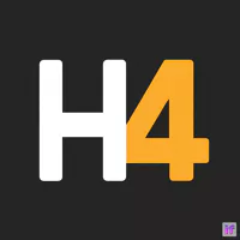
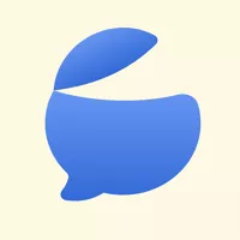
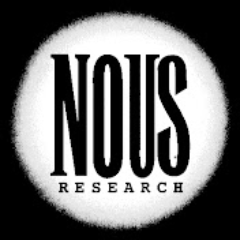
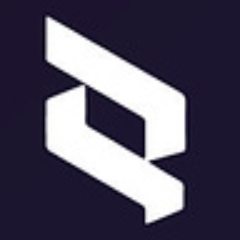
Other models include:
- GPT-4: Boasting an impressive context window of up to 128k, this model takes deep learning to new heights.
- Google Gemini Pro: Google's AI model designed for precision and depth in information retrieval.
- DALLE 3: Create stunning, high-resolution images from textual descriptions.
- Stable Diffusion: Generate images with a unique artistic flair, perfect for creative projects.
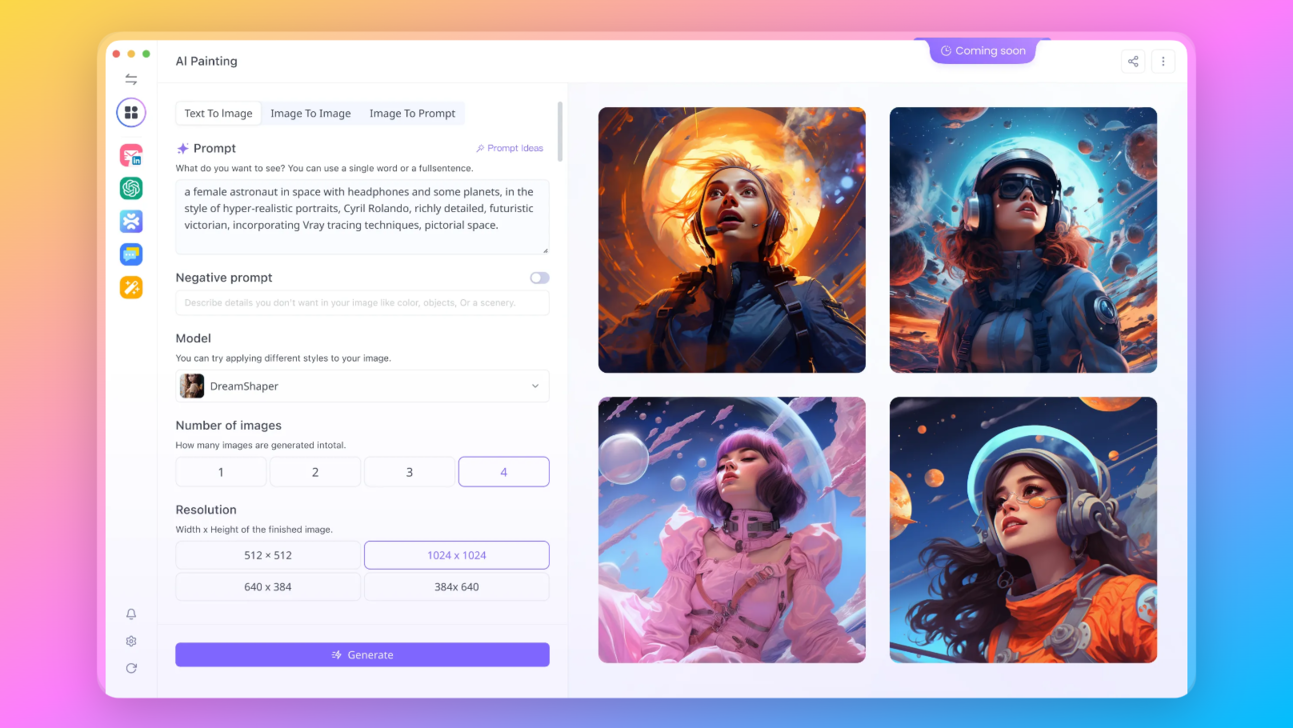
Interested in trying Open Chat-3.5-1210? Try it now at Anakin.AI!
The Training Process of WizardMath-7B-V1.1
The training of WizardMath-7B-V1.1 is a paradigm of complexity and sophistication. It’s constructed on a bedrock of novel machine learning techniques tailored specifically to grasp an
Conclusion
Incorporating these insights from actual users and developers provides a more nuanced view of SOLAR-10.7B-Instruct-v1.0. It highlights the importance of community feedback in evaluating and improving language models. This perspective underscores the model's strengths in detail-oriented instruction following and its potential for further refinement in areas like writing style and multi-turn conversation capabilities. Moreover, the community's active engagement in technical discussions, benchmarking, and skepticism towards performance claims reflects a mature, discerning approach to evaluating AI advancements.
from Anakin Blog http://anakin.ai/blog/solar-10-7b-instruct/
via IFTTT
No comments:
Post a Comment